Difference between revisions of "The Street View Text Dataset"
Line 24: | Line 24: | ||
=Current Version= | =Current Version= | ||
− | |||
− | |||
1.0 (also available from the [http://vision.ucsd.edu/~kai/svt/ Author's Web site]) | 1.0 (also available from the [http://vision.ucsd.edu/~kai/svt/ Author's Web site]) | ||
=Keywords= | =Keywords= | ||
+ | [[Image:StreetViewText_Sample.jpg|400px|thumb|right| Example images from the Street View Text dataset.]] | ||
OCR, Real Scene, Urban Scene, Scene Text, Word Spotting, Scene Text Recognition, Scene Text Detection, Scene Text Localization | OCR, Real Scene, Urban Scene, Scene Text, Word Spotting, Scene Text Recognition, Scene Text Detection, Scene Text Localization | ||
Latest revision as of 11:40, 27 October 2012
Datasets -> Datasets List -> Current Page
|
Contents
Contact Author
Kai Wang EBU3B, Room 4148 Department of Comp. Sci. and Engr. University of California, San Diego 9500 Gilman Drive, Mail Code 0404 La Jolla, CA 92093-0404 Email: k...@cs.ucsd.edu
Current Version
1.0 (also available from the Author's Web site)
Keywords
OCR, Real Scene, Urban Scene, Scene Text, Word Spotting, Scene Text Recognition, Scene Text Detection, Scene Text Localization
Description
The Street View Text (SVT) dataset was harvested from Google Street View. Image text in this data exhibits high variability and often has low resolution. In dealing with outdoor street level imagery, we note two characteristics. (1) Image text often comes from business signage and (2) business names are easily available through geographic business searches. These factors make the SVT set uniquely suited for word spotting in the wild: given a street view image, the goal is to identify words from nearby businesses. More details about the data set can be found in our paper, Word Spotting in the Wild [1]. For our up-to-date benchmarks on this data, see our paper, End-to-end Scene Text Recognition [2].
This dataset only has word-level annotations (no character bounding boxes) and should be used for
- cropped lexicon-driven word recognition and
- full image lexicon-driven word detection and recognition.
If you need character training data then you should look into the Chars74K and the ICDAR2003 and ICDAR2005 datasets.
Metadata and Ground Truth Data
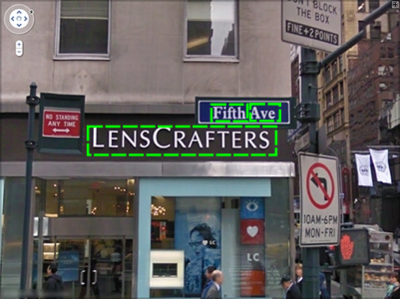
We used Amazon's Mechanical Turk to harvest and label the images from Google Street View. To build the data set, we created several Human Intelligence Tasks (HITs) to be completed on Mechanical Turk.
Harvest images
Workers are assigned a unique city and are requested to acquire 20 images that contain text from Google Street view. They were instructed to: (1) perform a Search Nearby:* on their city, (2) examine the businesses in the search results, and (3) look at the associated street view for images containing text from the business name. If words are found, they compose the scene to minimize skew, save a screen shot, and record the business name and address.
Image annotation
Workers are presented with an image and a list of candidate words to label with bounding boxes. This contrasts with the ICDAR Robust Reading data set in that we only label words associated with businesses. We used Alex Sorokin's Annotation Toolkit to support bounding box image annotation. For each image, we obtained a list of local business names using the Search Nearby:* in Google Maps at the image's address. We stored the top 20 business results for each image, typically resulting in 50 unique words. To summarize, the SVT data set consists of images collected from Google Street View, where each image is annotated with bounding boxes around words from businesses around where the image was taken.
The annotations are in XML using tags similar to those from the ICDAR 2003 Robust Reading Competition.
References
- Kai Wang, Boris Babenko and Serge Belongie, "End-to-end Scene Text Recognition", ICCV 2011, Barcelona, Spain (PDF). Galleries: ICDAR, SVT.
- Kai Wang and Serge Belongie, "Word Spotting in the Wild", ECCV 2010, Heraklion, Crete, Greece (PDF).
Download
Version 1.0
This page is editable only by TC11 Officers .